Geopolitics and Machine Learning: Can AI Be Used to Forecast IMF Programs?
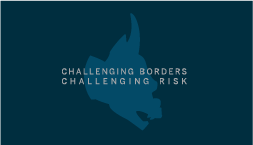
This interesting study from the IMF Working Paper series (1) applies state-of-the-art machine learning (ML) techniques to forecast IMF-supported programs, (2) analyzes the ML prediction results relative to traditional econometric approaches, (3) explores non-linear relationships among predictors indicative of IMF-supported programs, and (4) evaluates model robustness about different feature sets and time periods.
It was found that ML models consistently outperform traditional methods in out-of-sample prediction of new IMF-supported arrangements with key predictors that align well with the literature and show consensus across different algorithms.
The analysis underscores the importance of incorporating a variety of external, fiscal, real, and financial features as well as institutional factors and structural variables such as corruption and polity scores from the International Country Risk Guide (ICRG),
The findings also highlight the varying influence of data processing choices such as feature selection, sampling techniques, and missing data imputation on the performance of different ML models and therefore indicate the usefulness of a flexible, algorithm-tailored approach.
Additionally, the results reveal that models that are most effective in near and medium-term predictions may tend to underperform over the long term, thus illustrating the need for regular updates or more stable – albeit potentially near-term suboptimal – models when frequent updates are impractical.
Our data drives.
The PRS Group
https://www.imf.org/en/Publications/WP/Issues/2024/03/09/Predicting-IMF-Supported-Programs-A-Machine-Learning-Approach-545753
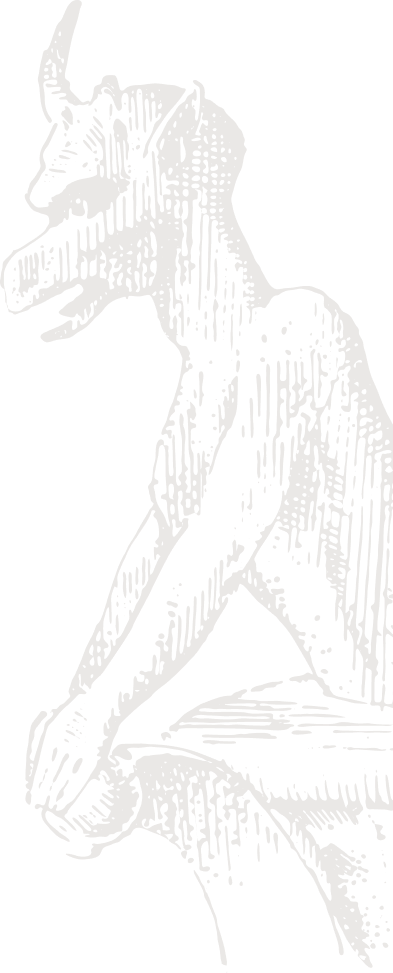
PRS INSIGHTS
Moving beyond current opinions, a seasoned look into the most pressing issues affecting geopolitical risk today.
EXPLORE INSIGHTS SUBSCRIBE TO INSIGHTS